- Introduction to Digital Biomarkers
Biomarkers refer to a group of features that can be assessed objectively. They are used for identification of regular biological states, pathogenic processes, or biological reactions that emerge as a result of being exposed to or receiving medical treatment.
Digitalization has affected healthcare services by expanding the sources and methods for assessing biomarkers and presenting a new set of measurable features.
This digital revolution enables digital technologies like mobile phones, wearables, sensors, and smart home appliances to make a novel group of biomarkers defined as “digital biomarkers” (Macias Alonso et al., 2024).
Digital biomarkers are defined as objective, quantifiable, physiological, and behavioral measures collected using digital devices that are portable, wearable, implantable, or digestible (Babrak et al., 2019). These biomarkers cover a wide range of health indicators, including heart rate variability (HRV), sleep patterns, step count, respiratory rate, and skin temperature.
Digital devices that people predominantly use for entertainment have begun to be viewed as potential sources. This data can be transformed into digital biomarkers (Motahari-Nezhad et al., 2022). The usage of digital tools in healthcare has rocketed up, especially with the COVID-19 outbreak, as new sensor-based devices and wearables altered how clinical information is collected, assessed, and stored. This shift has significantly influenced clinical decision-making. Today, digital biomarkers are getting more and more prevalent in regular treatment and studies across a number of medical specialties such as cardiology, oncology, neurology, and many more (Macias Alonso et al., 2024).
They assist with remote, continuous monitoring and help gather information that is not feasible to obtain in clinical settings. Additionally, they contribute clinical studies, enabling advanced treatment assessments and individualized care options.
Digital biomarkers improve diagnostic accuracy and enable ongoing health monitoring. Their ability to track disease progression and predict health risks is vital for precision medicine, minimizing clinical errors and supporting personalized clinical decisions (Motahari-Nezhad et al., 2022).
Image source: Statista – The Statistics Portal
The increasing importance of digital biomarkers is evident in their growing market value. Estimated at 1.8 billion dollars in 2021, the global digital biomarkers market is expected to grow to 16 billion by 2028 (Mikulic, 2024).
- Digital Biomarkers Relevant to Wellbeing
Digital devices are increasingly being used to gather information and diagnose various health conditions. By tracking physical and environmental data, such as heart rate, sleep cycle, blood values, skin temperature, energy expenditure, and many more, digital biomarkers provide a useful and detailed approach to assessing health and wellness.
Wearable devices become ideal tools for detecting mental health problems and exploring digital biomarkers since there is a profound relationship between daily activity, physical behavior, and mental well-being.
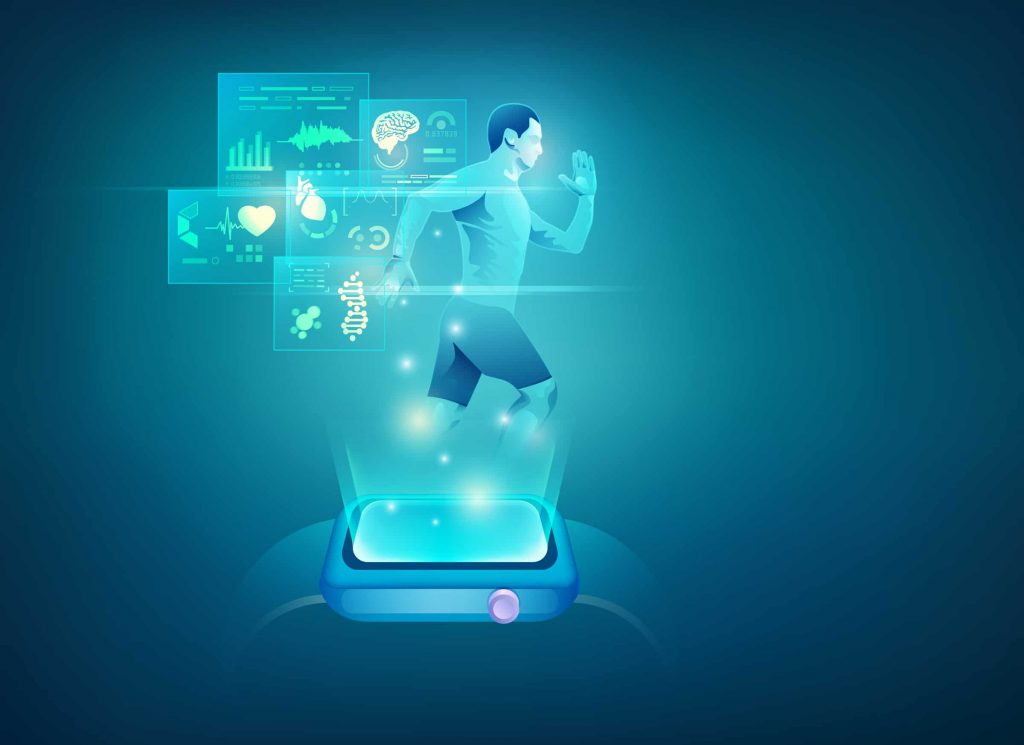
To illustrate, digital biomarkers that are collected by wearables could allow early and efficient depression screening. In a study, activity levels, sleep quality, and heart rate, which can act as “digital biomarkers” for depression, are monitored by wearables. According to its results, fluctuations in heart rate and disorganized circadian rhythms are the most dependable indicators of depression (Rykov et al., 2021).
Also, another instance of digital markers detecting diseases is their ability to recognize early symptoms of Alzheimer’s Disease (AD). Patients with Alzheimer’s disease usually exhibit deficiencies in motor functioning, which happen at least 10 years before impairment in cognitive symptoms is seen (Andreoletti et al., 2024). Additionally, emotional distress can be detected by evaluating differences in speech patterns derived from voice analysis through smartphones (Feel Therapeutics, 2024).
Besides these examples from mental health, wearable technology equipped with different kinds of sensors can identify many other diseases like hypertension, arrhythmias, sleep apnea, and chronic obstructive pulmonary disease (COPD).
These examples illustrate the transformative role of digital biomarkers in healthcare, providing continuous, personalized insights that facilitate proactive health management. Moreover, the employment of digital devices to detect symptoms and make predictions highlights a shift from traditional brain-focused approaches to a “digital gaze,” leveraging advanced technology for deeper insights (Mulinari, 2023).
- Digital Biomarkers in Skincare
Besides changing conditions and providing unique opportunities for mental and physical health, digital biomarkers also have contributed to the skincare industry. Both internal and external elements such as hydration, stressors in the environment, and stress and sleep quality have an impact on skin health. Digital biomarkers enable a full view of both internal and external elements by combining wearable devices and sensors. This facilitates individualized skincare treatments. There are some digital biomarkers that are intensively related to skin health.
- Skin Hydration and Temperature Monitoring
Assessing skin moisture and temperature can be seen as one of the most essential applications of digital biomarkers in the skincare field. Today, wearables with capacitive technology are able to detect the level of skin hydration while giving immediate information on the degree of moisture. One of these wearable devices that was introduced as an innovation reflecting the need for immediate and non-invasive hydration assessment, which is especially useful for individuals with greater physical activity, is HydroTrack (Belabbaci & Alam, 2024). To improve health tracking capacity, the system makes use of edge-based signal data processing and analysis approaches.
- Environmental Factors
Environmental factors, including air pollution, humidity, and UV radiation, largely affect skin health. Being exposed to UV radiation for longer durations is a major cause of aging of the skin and cancer, whereas polluted air induces oxidative damage and inflammation. Low humidity skin might result in dryness. In contrast, higher levels of humidity might cause oily skin and acne problems (WHO, 2023).
- Sleep Quality
High sleep quality is a crucial component of skin health. It contributes to the repair and renewal of skin cells, reaching its peak in deep sleep. Studies emphasize that poor sleep quality is linked with greater intrinsic aging, lessened functioning in the skin barrier, and dissatisfaction with appearance (Oyetakin-White et. al., 2014). Wearable devices such as mobile phones and smartwatches can assess sleeping patterns and imbalances, presenting insights about the connection between sleep quality and skin health. Digital biomarkers can motivate individuals to develop routines that enhance sleep quality for better skin health by detecting sleep defects.
- Stress and Skin Conditions
The level of stress considerably influences skin health, intensifying acne, eczema, and psoriasis. Stress detection systems are a growing trend now, enabling individuals to both understand and take care of their stress. There are several models introduced for predicting levels of stress from physiological elements such as electroencephalograms (EEG), heart rate variability (HRV), galvanic skin responses (GSR), blood pressure (BP), and behavioral elements like facial gestures, speech patterns, and posture. Both behavioral and physiological data can be gathered via smart devices. When sensor data and records obtained by smart devices are combined, stress can be anticipated. Wearables identifying indicative symptoms of greater stress can suggest relaxing strategies that may relieve stress-originate skin disorders. Incorporating biomarkers of stress with environmental and skin moisture data allows more extensive information to comprehend how stress and external variables affect skin health (Haque et al., 2023).
- AI and Digital Biomarkers
The skincare and wellbeing landscape enables advancements in personalized diagnostics and treatment solutions revolutionized by the combination of biological markers and artificial intelligence. AI and machine learning are very important keys to interpreting data from biological markers used on skin and hair. Artificial intelligence trained on the data of many biological markers offers data-driven decisions to the field.
The data given to AI consists of various digital biomarkers such as skin tone, melanin index, trans-epidermal water loss, evenness, roughness, elasticity, and fluorescence. Spectrometers, colorimeters, and skin conductometers are the tools that collect these digital biomarkers, and then the data is transformed into numerical data for AI to train.
To illustrate, AI-enabled algorithms can evaluate both optical and mechanical properties of the skin. This allows precise assessment of many skin conditions, such as acne, aging, and the degree of hydration. One of the significant instances is the usage of UV-induced skin fluorescence to detect porphyrin level, which is a substantial biomarker for acne. Nonetheless, individual differences have an impact on the intensity and spectral composition of fluorescence. This emphasizes the problem of embedded biases in AI systems (Chekanov et al., 2024).
The implementation of AI systems in the skincare field has altered the whole value chain, beginning with basic research to individualized services. Sophisticated ML models analyze extensive, multifaceted data for offering accurate skin treatments and product suggestions that are unique to the needs of each person. This technique assures a more relevant and result-oriented relationship with consumers, improving their well-being mentally and physically (Georgievskaya et al., 2023).
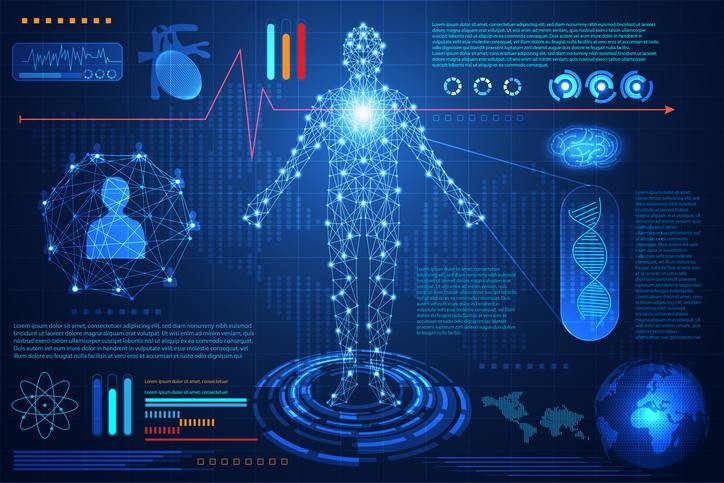
In spite of the opportunities that AI brings, there are also some challenges to relying on AI and digital biomarkers. The main considerations are as follows: biases in the collection of data, annotation, and analysis. Lots of data sets employed for training algorithms in skincare have inherent biases, underrepresenting different groups of pigmentation, age, and ethnicity. This limits the applicability.
Moving to monitoring of AI systems post-production is also crucial, as ongoing social and biological shifts can cause “concept rift.” This term refers to the evolution of the relationship between markers and results in time. Continuous improvements and calibration of AI systems are a necessity to sustain precision, safety, and inclusion. Furthermore, AI must incorporate changing trends in skincare and consumer demands (Arya et al., 2023).
AI-driven systems in skincare are not beneficial only for improving assessment but also foster individualized wellness experiences. These technologies can generate personalized recommendations by integrating digital biological markers with advanced AI algorithms, aiding people to achieve ideal skin health. Plus, they represent society’s ideals by exposing embedded biases in data and urging equitable treatment for diverse populations (Georgievskaya et al., 2023).
To sum up, the integration of AI algorithms with digital biomarkers constitutes a significant evolution in skincare and wellness. These algorithms provide a broader understanding of skin health while resolving ethical and inclusion concerns. AI-driven skincare recommendations have the potential to help people on their quest to healthier, more confident lifestyles through ongoing innovation and tracking.
References
Andreoletti, M., Haller, L., Vayena, E., & Blasimme, A. (2024). Mapping the ethical landscape of Digital Biomarkers: A scoping review. PLOS Digital Health, 3(5). https://doi.org/10.1371/journal.pdig.0000519
Arya, S. S., Dias, S. B., Jelinek, H. F., Hadjileontiadis, L. J., & Pappa, A.-M. (2023). The convergence of traditional and digital biomarkers through AI-assisted biosensing: A new era in translational diagnostics? Biosensors and Bioelectronics, 235, 115387. https://doi.org/10.1016/j.bios.2023.115387
Belabbaci, N. A., & Alam, M. A. U. (2024). HydroTrack: Spectroscopic Analysis Prototype Enabling Real-Time Hydration Monitoring in Wearables. arXiv preprint arXiv:2407.11997.
Chekanov, K., Danko, D., Tlyachev, T., Kiselev, K., Hagens, R., & Georgievskaya, A. (2024). State-of-the-art in skin fluorescent photography for cosmetic and skincare research: From molecular spectra to AI Image Analysis. Life, 14(10), 1271. https://doi.org/10.3390/life14101271
Feel Therapeutics. (2024, January 19). Digital Precision Mental Health: The role of wearable technology in objective monitoring. Digital Precision Mental Health: The Role of Wearable Technology in Objective Monitoring. https://www.feeltherapeutics.com/post/wearable-technology-in-mental-health
Georgievskaya, A., Tlyachev, T., Danko, D., Chekanov, K., & Corstjens, H. (2023). How artificial intelligence adopts human biases: The case of cosmetic skincare industry. AI and Ethics. https://doi.org/10.1007/s43681-023-00378-2
Haque, Y., Zawad, R. S., Rony, C. S., Al Banna, H., Ghosh, T., Kaiser, M. S., & Mahmud, M. (2023). State-of-the-art of stress prediction from heart rate variability using artificial intelligence. Cognitive Computation, 16(2), 455–481. https://doi.org/10.1007/s12559-023-10200-0
Macias Alonso, A. K., Hirt, J., Woelfle, T., Janiaud, P., & Hemkens, L. G. (2024). Definitions of digital biomarkers: A systematic mapping of the biomedical literature. BMJ Health & Care Informatics, 31(1). https://doi.org/10.1136/bmjhci-2023-100914
Mikulic, M. (2024, June 19). Publications on AI used for understanding diseases: Share by sub-use Case worldwide 2018-2022. Statista. https://www.statista.com/statistics/1428377/publications-on-ai-used-for-understanding-diseases-worldwide/
Motahari-Nezhad, H., Fgaier, M., Mahdi Abid, M., Péntek, M., Gulácsi, L., & Zrubka, Z. (2022). Digital Biomarker–based studies: Scoping Review of Systematic Reviews. JMIR mHealth and uHealth, 10(10). https://doi.org/10.2196/35722
Mulinari, S. (2023). Short-circuiting biology: Digital phenotypes, digital biomarkers, and shifting gazes in psychiatry. Big Data & Society, 10(1). https://doi.org/10.1177/20539517221145680
Oyetakin-White, P., Suggs, A., Koo, B., Matsui, M. S., Yarosh, D., Cooper, K. D., & Baron, E. D. (2014). Does poor sleep quality affect skin ageing? Clinical and Experimental Dermatology, 40(1), 17–22. https://doi.org/10.1111/ced.12455
Rykov, Y., Thach, T.-Q., Bojic, I., Christopoulos, G., & Car, J. (2021). Digital biomarkers for depression screening with wearable devices: Cross-sectional study with machine learning modeling. JMIR mHealth and uHealth, 9(10). https://doi.org/10.2196/24872